Agenda
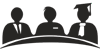
Soutenance de thèse de Yuding WANG
Vendredi 15 décembre 2023, à 14h, à l’Enssat (salle 020 G), LannionYuding WANG soutiendra sa thèse de doctorat intitulée : « Partitioning of large hyperspectral image data streams », sous la direction de Kacem Chedhi (PR, IETR - équipe Multip Lannion / Université de Rennes, directeur de travaux).
Abstract:
With the development of automated and optimized decision-making systems, large data stream partitioning, which does not rely on training samples, has attracted more and more attention. In the stat-of-the-art, a majority of data stream partitioning methods are parametric which require the specification of one or more user-defined parameters and/or the number of classes before the partitioning process. Indeed, in some practical applications, it is difficult to know the prior information of the dataset in advance. Therefore, our research focuses on the development of unsupervised and non-parametric method which is easy to apply by users, benefiting from the fact that it eliminates the need for prior information and obviates the requirement for empirical parameters tuning.
In this way, the developed method can autonomously estimate the number of classes and partition the data stream. It must also be efficient to partition large and high spatial and spectral dimensional data streams, especially hyperspectral data streams.
Our proposed method was assessed on real-world and synthetic databases. According to several objective evaluation criteria, it performs better than the compared parametric partitioning methods and a method using active learning..